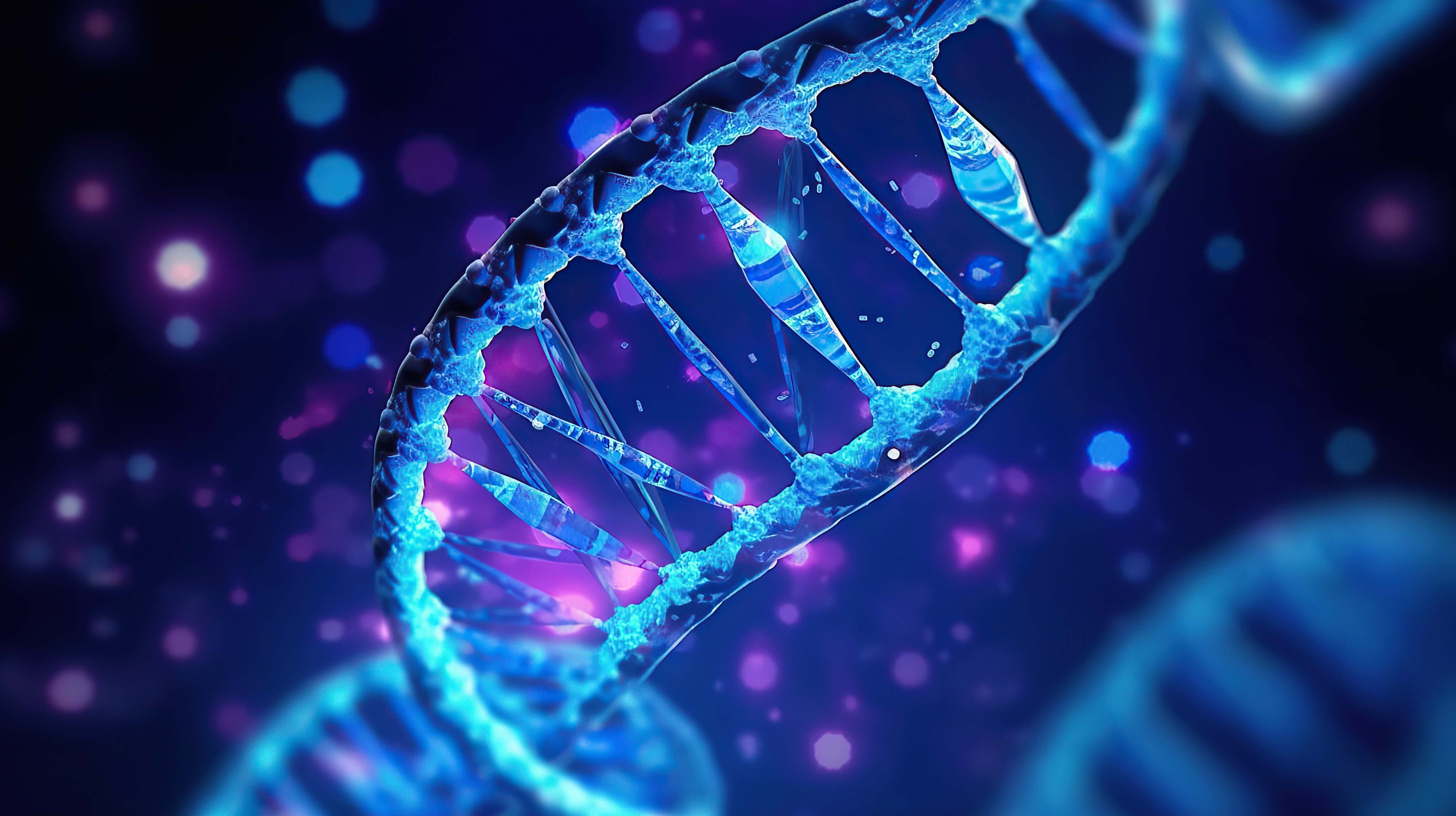
Drop off your CV/Resume
We'd love to hear from you. Send us your CV/Resume and one of our team will be in touch.
There was once a time when AI was the stuff of science fiction. Today, it’s a part of ...
There was once a time when AI was the stuff of science fiction. Today, it’s a part of our reality that we can’t seem to escape. But fear not, it seems we might have just found its most exciting use yet…
It’s no secret that drug development is lengthy, expensive, labor-intensive, and often unsuccessful. In fact, of the 10,000 molecules initially screened, only around 10 make it into clinical trials, and even then, while many still fail, for those that don’t, it takes around 10-12 years to bring a drug to market, with R&D costing around $2.2 million per drug.
Not just that, over the last decade, the expected ROI has declined from 10% to just 2% per treatment. That means that today, in order for the industry to remain lucrative, it’s absolutely essential that we find more efficient and cost-effective ways to bring new drugs to market.
Enter AI.
As we wade through a more digitalized reality, within Life Sciences, AI application is being utilized right across the value chain. For drug development in particular, the technology is being leveraged to crawl gigantic datasets to enable faster and cheaper discovery of new molecules.
Essentially, with the use of data science and machine learning, AI is cross-referencing published scientific literature with alternative information sources, such as clinical trial information, public databases, conference abstracts, and unpublished data dating back decades.
With 38% of biopharmas using AI daily, this has already resulted in the delivery of new candidate medicines, in some cases in months rather than years, totally revitalizing R&D productivity.
That’s because instead of researchers having to create big batches of molecules and manually testing them all in the hope that they have certain features, they’re now able to begin with a wishlist of mathematically encoded characteristics. They then produce designs for molecules that have those desired properties at the push of a button, flipping early phase development on its head by speeding up cycles of exploration.
AI also has the ability to predict how potential drugs might behave in the body and discard dead-end compounds before they’ve even left the computer, which again cuts down some of the painstaking lab work for researchers.
Today, over two dozen drugs (and counting!) that have been developed with AI assistance are already in or are about to enter clinical trials. So, what diseases are being targeted with the use of AI?
It’s been decades since new drugs have been developed to treat bacterial infections, and as bacterium has evolved, they’ve become increasingly resistant to current antibiotics used to treat them.
In fact, because it’s become such an urgent healthcare need, with over 1 million people per year dying from infections fighting antibiotic treatment, the World Health Organization drew up a list of bacteria resistant to multiple different types of antibiotics to encourage R&D activities for new strains.
Within that list, one of the most challenging species of bacteria, Acinetobacter Baumanii, which infects the blood system, urinary tract, other wounds on the body and causes pneumonia, has been identified as one of three ‘superbugs’ deemed a critical threat.
The reason why is due to its ability to shake off vast amounts of antibiotics. What’s more, this type of bacteria lives on surfaces and medical equipment, causing major problems across hospitals and care homes.
To beat this life-threatening infection, researchers at McMaster University have trained AI to find a new antibiotic, taking thousands of drugs with known chemical structures and testing them on the bug with the hope of slowing it down or even killing it.
In less than two hours, the AI technology produced a shortlist of effective compounds. From there, the team of researchers tested 240 compounds and found nine potential antibiotics that could stop the bacteria.
In breakthrough trials, the antibiotic Abaucin showed its ability to treat infected wounds in mice and kill the bacteria in samples from patients. These promising results will lead the researchers to perfect the drug and enter it into clinical trials to test how it works on a larger scale.
As it stands at the moment, with the promise of AI, the team expects the first AI-assisted antibiotics to be available to the public by 2030.
The oncology sector has been pursuing personalized treatments for some time, but Exscientia, a UK-based pharmatech, is looking at individualized cancer therapies through a new lens.
By implementing AI matchmaking technology into their clinical trials, the company is pairing patients with the precise drugs they need to account for the subtle biological differences between individuals.
To do so, they take a small tissue sample from each patient, then divide the sample (including both normal and cancer cells) into over 100 fragments and expose each of them to a different combination of drugs.
From there, machine learning models trained to identify small changes in cells test dozens of treatments simultaneously to find the perfect match for each patient and their particular disease. This means that researchers can carry out exhaustive searches for the right drug for each individual.
To this day, the company has taken tissue samples from dozens of cancer patients who’ve undergone at least two failed courses of chemotherapy and evaluated the effects of 139 existing drugs on their cells. The results show that altogether, they’ve been able to identify a drug that worked for more than half of the patients.
But Exscientia isn’t stopping there. On top of pairing patients up with existing drugs, they’re utilizing machine learning to design new ones too! Thus far, the company already has drugs designed with AI in clinical trials and is about to submit two more.
Commenting on their success, CEO, Andrew Hopkins says, “If we were using a traditional approach, we couldn’t have scaled this fast.”
Now the company plans on using AI technology to further shape its approach to drug development, with Hopkins commenting, “Instead of starting with a model of a disease, we can start with the tissue from a patient. The patient is the best model.”
IPF is a chronic long-term disease that affects five million people per year, stiffening their lung tissues and possibly even leading to death. Unfortunately, there is no known cure for IPF, but thanks to Insilico Medicine, it looks like there’s promise on the horizon…
Earlier this year, Insilico made headlines after beginning Phase II clinical trials for their new IPF drug developed entirely by AI, from target discovery to drug design.
After making use of natural language processing and training AI to understand the different types of fibrosis, the biotech found the technology could mine massive data sets in search of the protein causing fibrosis and potential targets.
Once identified, another type of AI called Generative Adversarial Network (GAN) then detected a molecule with the potential to become an effective drug against IPF. At this stage of R&D, GAN essentially pits the two different types of AI against one another – one generating suggestions and the other critiquing those suggestions until the most reliable candidate is found.
From there, the company used AI to monitor the effects of the drug on eight volunteers. After promising results, their Phase I trials tested the drug on a larger study group of 78 participants, half of whom were given increasing dosages, to determine how the human body reacts to the drug’s molecules.
The results found that the patients reacted well to the treatment with no serious or adverse side effects. Now the company has entered Phase II trials to further test the safety, tolerability, pharmacokinetics, and efficacy of a 12-week oral dosage of the drug.
This news is a complete breakthrough for sufferers of IPF, but it also highlights just how important and invaluable AI can be in developing new therapies. So far, Insilico’s research has taken two and a half years and $3.4 million – a fraction of the time and cost traditional drug development usually takes, which also suffers from a 90% failure rate.
Looking forward, the biotech is also in the process of researching innovative AI-assisted drugs for cancer, immunity, central nervous system diseases, and age-related diseases.
The aforementioned examples only reiterate the value of AI, not to compete with human endeavors but to elevate them. So while we wait for the first batch of AI-designed drugs to make their way through clinical trials, it’s fair to say that AI advancements signal a dawn for drug development, providing us with a higher level of knowledge on disease mechanisms, increasing the number of treatments available, speeding up the availability of drugs capable of treating specific diseases, and dramatically improving the lives of millions of patients.
Want to find out in what other capacities AI is transforming Life Sciences? Subscribe to our newsletter, where we’ll be covering the effects of AI on other aspects of the industry we wholeheartedly support.